artificial intelligence, Digital Forensics
Deepfakes: Much More Than Meets The Eye
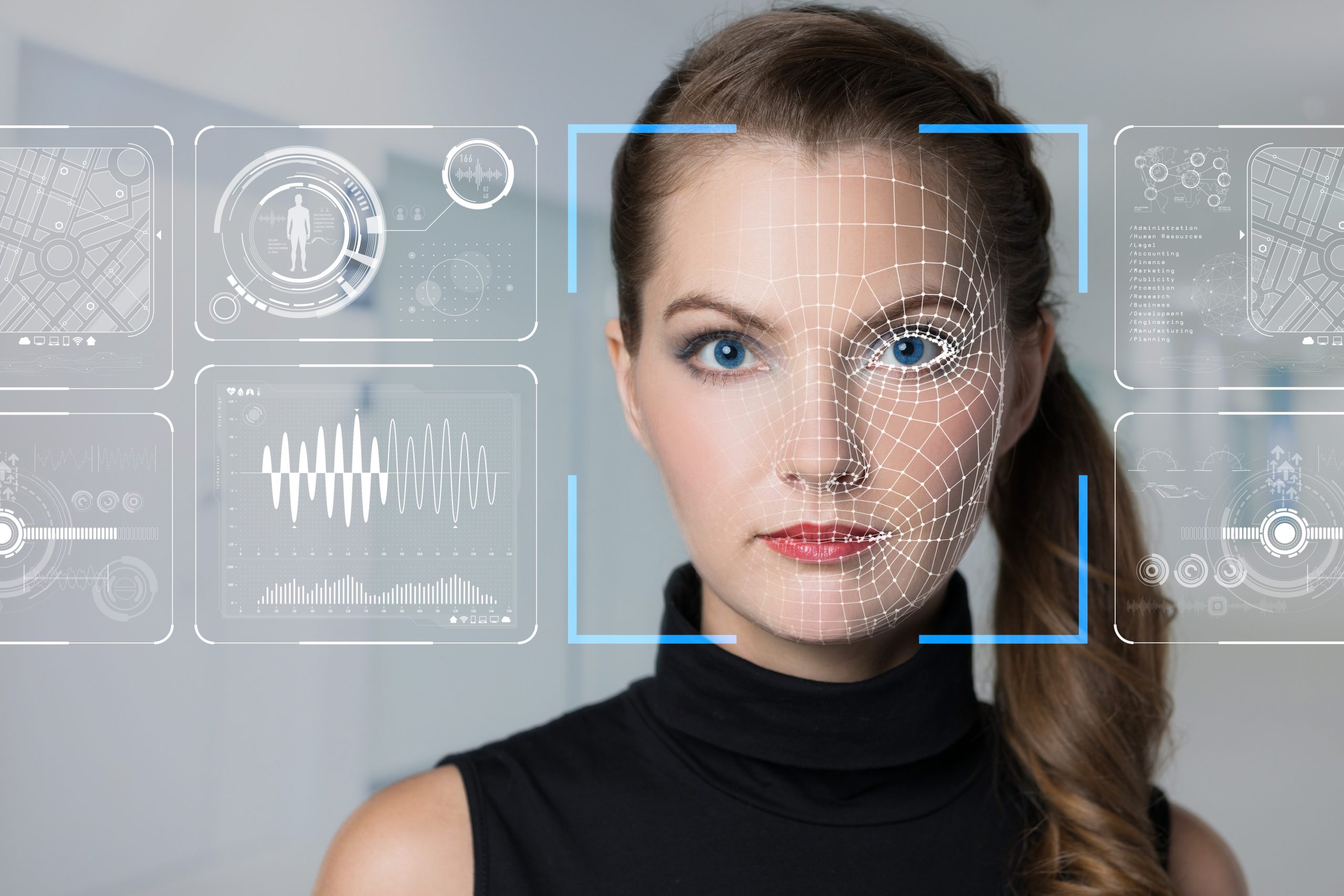
Written By
Falsified news, media, and online digital content is a concern. Technological advancements allowing realistic look-alike video and images, also known as “Deepfakes” are posing problems for those tasked with identifying original versus simulated content. To detect a “Deepfake” it is imperative to understand how the content is being created. While not always the case, these simulated videos and images often have use cases of fraud, defamation, spreading misinformation and impersonation.
As artificial intelligence and machine learning advances so will the use of deepfakes to assist the creators with reaching their intended goal, of defaming or slandering their target. A deepfake is a digitally altered video, audio or image file which depicts an individual(s) in a negative manner and is often used to spread false information about a person or topic. There are several methods of creating a Deepfake. You need an original video, audio clip or image to use as the basis for the simulated Deepfake. Next, you need similar video, audio or image content of the same subject to help build the new simulated Deepfake. Essentially, you are taking a video or image, and then taking similar content to make the original video appear to be different than it truly is, using various similar clips.
This is done using programs and applications that use autoencoders to facilitate face-swapping techniques. However, Deepfakes are not new to the world of misinformation. In 1997 a program called Video Rewrite altered existing videos and made it appear the subject depicted in the video was saying things they never actually did. Video Rewrite was the first program to ever automate facial reanimation. Today, there are many programs and applications, like DeepSwap, FaceSwap and TalkingFaces, whose sole purpose is to create deepfakes.
Detecting a deepfake with the naked eye is particularly challenging, however, there are several things to look for when reviewing suspected fraudulent videos. One thing to look for is when any coughing, sneezing or another involuntary action does not match the actual actions or audio of the video. For example, the subject in the video may be casually walking and the audio contains a coughing sound. The video will then depict a very unnatural coughing motion by the subject in the video. Also, pay close attention to the eyes of the main subject in the video. The head movements will not move properly, and facial expressions will not coincide with the actions of the video. Further, the posture of the main subject will not align with their usual posture and will not look like a natural body movement. There may also be audio which does not belong with the video or varies greatly in volume while viewing the video. Lastly, the video’s audio will not align with the lips of the subject. Utilizing these key points will help with detecting deepfakes. However, several programs are being developed to automate the process.
One of the programs designed to help identify deepfakes is FakeCatcher by Intel, which uses AI (artificial intelligence) to identify deepfake content and claims 96% accuracy. The program runs on an Intel server and uses multiple Intel designed tools to evaluate the content via a web-based platform. What makes FakeCatcher different from other deepfake detectors, is that most look at the video’s raw data to attempt to determine if it was manipulated in any way. FakeCatcher evaluates the data at the pixel level and looks for signs of human blood flow. A fake video shows numerous inconsistencies in facial blood flow, allowing the software to identify that the video is not authentic. There are many use cases for such a program and these cases will only grow as the technology enabling Deepfakes evolves.
As deepfake technology not only grows in popularity but also in sophistication, so should the techniques used to detect it. Social media is becoming omnipresent in our daily lives, which is making what people view on it even more important. Having the ability to quickly identify, report, and remove fake content from whatever platform it may infiltrate will have a profound effect on society. A single approach to detection is not effective or prudent. A toolbox approach is best utilized to achieve this objective. What tools you use to fill your toolbox will be entity / task specific, but keeping a constant eye on the ever-changing landscape of fake information is the first step in prevention.
Additionally, part of what make deepfakes so hard to detect is that the machine learning they employ is constantly evolving. This learning is done using various types of networks collaborating with each other. Two of these types of networks are neural networks and generative adversarial networks (GANs). In an upcoming post we will take a closer look at these networks along with their related technologies and how they work together to create ever more convincing deepfakes.
About Capsicum:
Capsicum was founded in 2000 within the law firm of Pepper Hamilton, LLP. (now Troutman Pepper Hamilton Sanders LLP.) Charged with providing technology consulting support to their clients, we soon realized that the need to understand, collect, and forensically analyze digital data went far beyond what we were handling. We began our journey as general technologists, but quickly became specialists in digital forensics. Our areas of expertise soon evolved and expanded into forensic investigations, cybersecurity, discovery, electronic and paper recovery, security, regulatory compliance, and incident response retainers. In 2002, Capsicum became an independent consulting company that focuses on these core services. Employing high-caliber experts and a unique understanding of data, technology, and the law, we support organizations that need technological proficiency to run their companies and when they come face-to-face with difficult tech, legal, and regulatory situations. Capsicum is located in Philadelphia, PA; New York, NY; Fort Lauderdale, FL; Dallas, TX; and Los Angeles, CA.